There’s a war between two visions of how the ubiquitous AI assisted future will be rendered: on the cloud or on the device. And as with any great drama it helps the story along if we have two archetypal antagonists. On the cloud side we have Google. On the device side we have Apple. Who will win? Both? Neither? Or do we all win?
If you would have asked me a week ago I would have said the cloud would win. Definitely. If you read an article like Jeff Dean On Large-Scale Deep Learning At Google you can’t help but be amazed at what Google is accomplishing. Impressive. Wide ranging. Smart. Systematic. Dominant.
Apple has been largely absent from the trend of sprinkling deep learning fairy dust on their products. This should not be all that surprising. Apple moves at their own pace. Apple doesn’t reach for early adopters, they release a technology when it’s a win for the mass consumer market.
There’s an idea because Apple is so secretive they might have hidden away vast deep learning chops we don’t even know about yet. We, of course, have no way of knowing.
What may prove more true is that Apple is going about deep learning in a different way: differential privacy + powerful on device processors + offline training with downloadable models + a commitment to really really not knowing anything personal about you + the deep learning equivalent of perfect forward secrecy.
Photos vs Photos
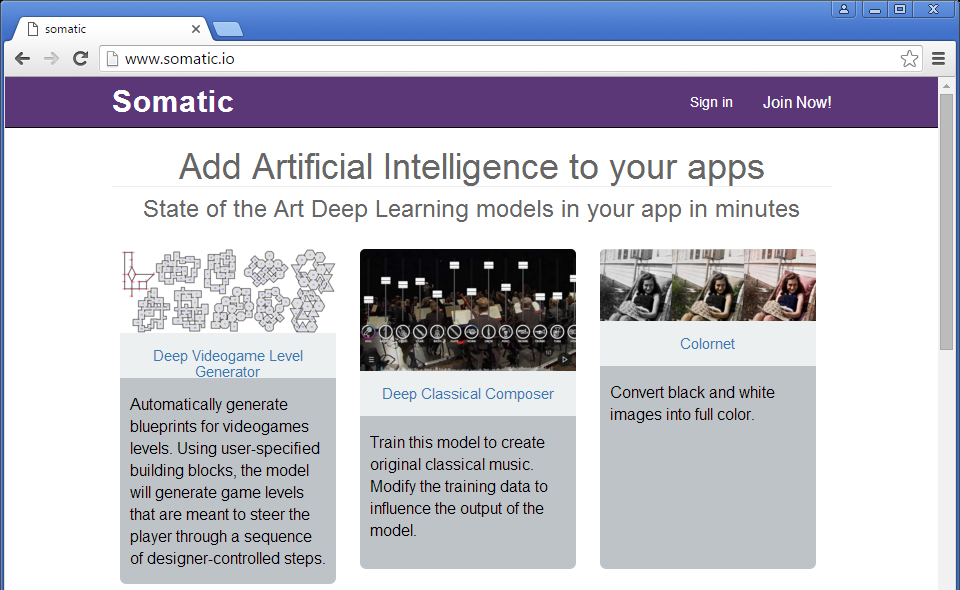
Link: http://www.somatic.io/
Pricing:
- Development $199/mo
- Professional $499/mo
- Production $999/mo
- Enterprise (contact for pricing)
Currently 23 Models:
- Deep Videogame Level Generator – Automatically generate blueprints for videogames levels. Using user-specified building blocks, the model will generate game levels that are meant to steer the player through a sequence of designer-controlled steps.
- Deep Classical Composer – Train this model to create original classical music. Modify the training data to influence the output of the model.
- Colornet – Convert black and white images into full color.
- Deep 3D Plants – Automatically generate 3D plants of different kinds, shapes, and sizes.
- inception-prebuilt – This model allows a data scientist to customize which level in an ANN’s (artificial neural network) hierarchy structure to enhance. Lower levels enhance low level features such as lines and basic shapes. Higher levels enhance actual structures suc…
- neuraltalk2-demo – This vision-to-language model analyzes the contents of an image and outputs an English sentence about what it sees. The model was trained using “storyable” events from Flickr and captions that were generated by crowdsourcers on Amazon’s Mechanical…
- char-rnn-ted – his code implements multi-layer Recurrent Neural Network (RNN, LSTM, and GRU) for training character-level language models. In other words, the model takes one text file as input and trains an RNN that learns to predict the next character in a s…
- DCGAN-faces – A neural network that is able to synthesize fake images based off seeing similar images. This particular model generates faces.
- deep3d – As 3D movies and Virtual Reality become more mainstream, the market for 3D content will grow at an exponential rate. However, producing 3D videos is a challenging and expensive process. This model allows for the conversion of 2D images into stereo…
- Caption to Image Generator – This model generates a strip of images that illustrate a caption.
- GRAN Image Generator – This model can be fed a large image dataset of objects such as faces, cars, chairs, etc. Using the data, it will generate a canvas of brand new objects in this class.
- English to Old English Translator – Translate old English (like the works of Shakespeare) into Modern English and vise versa.
- Context Encoder – This algorithm uses context clues to predict and render a missing part of an image.
- Deep Go – Train bots to play the classic board game Go. Interact with your bot through a web browser.
- Autotag Movie Clips – This model automatically indexes the contents of each frame of a video so that you can search and filter video scenes by objects, setting, actors, and more. Save time searching through hundreds of hours of archived footage by instantly queueing th…
- reverse image search – Give an image, return a list of all similar images from your database. We use a deep neural network architect with 8 layers to be able to compare images.
- Neural Doodle – This model allows you to transfer the style of one image on to another image. By creating a simple doodle map, you can specifically tell the AI how you want the style to be transferred to your target image.
- neural-style-demo – Transfer the style from one image and apply it to a target image. Imagine a self portrait in the style of Van Gogh’s [sic] Starry Night.
- GRAN Cat Generator – This model can be fed a large image dataset of objects such as faces, cars, chairs, etc. Using the data, it will generate brand new objects in this class. This particular model generates cute cats.
- neural-storyteller – This model analyzes an image and produces a story about what it sees. The model can be trained with various data sets in order to alter the story’s voice, tone, and word choice. This particular model was trained using romance novels but you could …
- Video Inception – This model allows for deep dreaming in videos. Train the model to “hallucinate” objects in each frame of a video.
- ClearText – This text editor only allows you to use the 1000 most common words in the English language, forcing you to write more clearly.
- image classifier – given any image, tell you if it belongs to a certain class.
Deep Learning Code Tutorials
The Deep Learning Tutorials (DeepLearning.net) are a walk-through with code for several important Deep Architectures (in progress; teaching material for Yoshua Bengio’s IFT6266 course).
Unsupervised Feature and Deep Learning
Stanford’s Unsupervised Feature and Deep Learning (UFLDL) tutorial has wiki pages and Matlab code examples for several basic concepts and algorithms used for unsupervised feature learning and deep learning.
Adapted from http://deeplearning.net/reading-list/tutorials/ on 4/4/15
Software links
C++ or C++/CUDA:
- Cuda-Convnet – A fast C++/CUDA implementation of convolutional (or more generally, feed-forward) neural networks. It can model arbitrary layer connectivity and network depth. Any directed acyclic graph of layers will do. Training is done using the back-propagation algorithm.
- CXXNET – CXXNET is fast, concise, distributed deep learning framework based on MShadow. It is a lightweight and easy extensible C++/CUDA neural network toolkit with friendly Python/Matlab interface for training and prediction.
- Eblearn is a C++ machine learning library with a BSD license for energy-based learning, convolutional networks, vision/recognition applications, etc. EBLearn is primarily maintained by Pierre Sermanet at NYU.
- MShadow – MShadow is a lightweight CPU/GPU Matrix/Tensor Template Library in C++/CUDA. The goal of mshadow is to support efficient, device invariant and simple tensor library for machine learning project that aims for both simplicity and performance. Supports CPU/GPU/Multi-GPU and distributed system.
- The CUV Library (github link) is a C++ framework with python bindings for easy use of Nvidia CUDA functions on matrices. It contains an RBM implementation, as well as annealed importance sampling code and code to calculate the partition function exactly (from AIS lab at University of Bonn).
Java:
- neuralnetworks is a java based gpu library for deep learning algorithms.
LUSH:
- The LUSH programming language and development environment, which is used @ NYU for deep convolutional networks
- Eblearn.lsh is a LUSH-based machine learning library for doing Energy-Based Learning. It includes code for “Predictive Sparse Decomposition” and other sparse auto-encoder methods for unsupervised learning. Koray Kavukcuoglu provides Eblearn code for several deep learning papers on this page.
Matlab/Octave:
Python:
- cudamat is a GPU-based matrix library for Python. Example code for training Neural Networks and Restricted Boltzmann Machines is included.
- Gnumpy is a Python module that interfaces in a way almost identical to numpy, but does its computations on your computer’s GPU. It runs on top of cudamat.
- 3-way factored RBM and mcRBM is python code calling CUDAMat to train models of natural images (from Marc’Aurelio Ranzato).
- mPoT is python code using CUDAMat and gnumpy to train models of natural images (from Marc’Aurelio Ranzato).
- Theano – CPU/GPU symbolic expression compiler in python (from LISA lab at University of Montreal)
- Pylearn2 – Pylearn2 is a library designed to make machine learning research easy.
Miscellaneous:
- Nengo-Nengo is a graphical and scripting based software package for simulating large-scale neural systems.
- RNNLM– Tomas Mikolov’s Recurrent Neural Network based Language models Toolkit.
- Torch – provides a Matlab-like environment for state-of-the-art machine learning algorithms in lua (from Ronan Collobert, Clement Farabet and Koray Kavukcuoglu)
Categorized/organized version of http://deeplearning.net/software_links/ as of 4/4/15
Computing, technology, general information